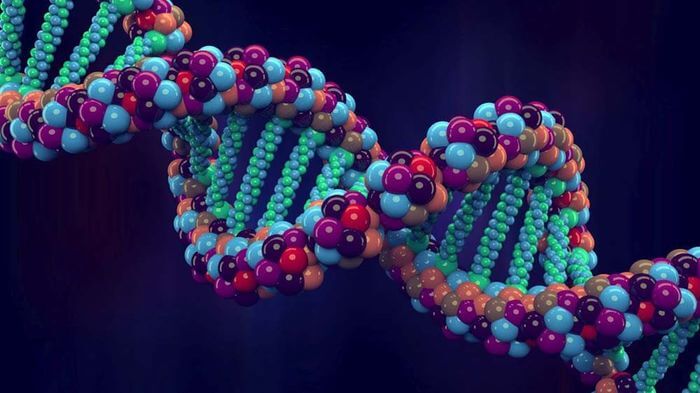
ML Framework for Characterizing a Novel Epigenome Modification Using DNA-Sequencing Assays
Motivation: While the human genome project sequenced the human genome in 2003, understanding the function of the genome beyond protein coding regions has been challenging and has not properly allowed us to leverage this data as well as we can for biomedical research.
Approach: Team built machine learning models that helped create functional annotation of human genome: which regions of the DNA are relevant for DNA - protein binding, which regions are regulators of gene expression, which regions govern such regulatory programs relevant in health, disease, development and adulthood, are all incredibly important questions to use machine learning models to understand.
Our machine learning frameworks (support vector machine, conditional random fields, random forests, hierarchical hidden markov models) were built with the purpose of functionally annotating human DNA in the context of DNA evolution, analysis of human cell line data, and other mammalian datasets.
Machine learning toolkits that we built have been deployed in multiple research laboratories to assist principal investigators to perform data analysis of their high throughput assays. Work performed by these laboratories have led to high visibility publications in top journals like Nature and Cell.